Why in the News?
Recently, the Cos-it-FloWs, a new system that collects hyper-local data for flood forecast launched in the flood-prone Periyar and Chalakudi river basins was launched in Kerala.
About CoS-it-FloWS
- CoS-it-FloWS (Community-Sourced Impact-based Flood Forecast and Early Warning System) is a project run by Equinoct, a Kochi-based community-sourced modelling solution provider.
- Recognized by UNICEF's Climate Tech Cohort, it uses 100 rain gauges installed across Ernakulam, Idukki, and Thrissur.
- Data on rainfall, river, tidal and groundwater levels that are collected primarily by students, women, and youth at the household level is then analyzed and visualized through Insight Gather, a web portal to host the impact-based forecasts in the pilot basins.
- The idea is to plug gaps in government data and to scale up the project with greater community participation by collecting hyper-local data for fighting natural disasters.
About hyperlocal weather forecasting
- Definition: Hyperlocal weather forecasting is a specialized form of meteorology that pinpoints weather conditions to extremely localized areas.
- Current forecasting level: Currently the Indian Meteorology Department (IMD) issues weather forecasting for district level.
- The forecast and warnings issued from National Weather Forecasting Centre (NWFC) are in the subdivisional scale for the country as a whole whereas the same from State WFC (SWFC) are in the district scale for the state concerned.
- Need for Hyperlocal forecasting: In tropical countries like India, weather variability is inherently higher. Hence hyperlocalized weather forecasting is needed for better utility.
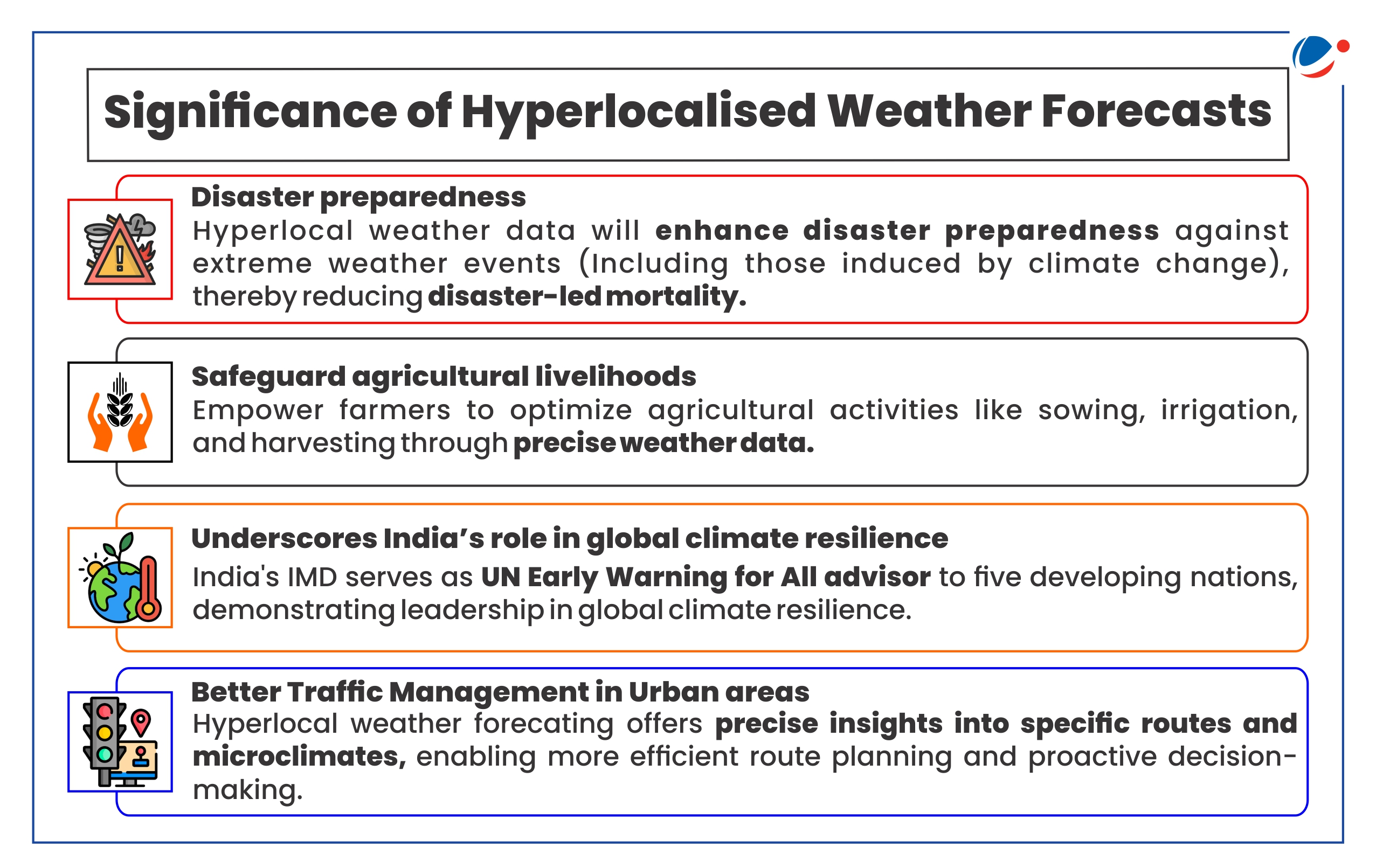
Key Challenges in hyperlocal weather forecasting
- Outdated prediction models: Currently, most of the prediction software used in forecasting are based on the Global Forecasting System (GFS) and Weather Research and Forecasting (WRF) Models, both of which are not the most modern.
- Lack of weather monitoring ground stations: Currently, IMD operates around 800 automatic weather stations (AWS), 1,500 automatic rain gauges (ARG) and 37 doppler weather radars (DWR).
- This is against the total requirements of more than 3,00,000 ground stations (AWS/ARG) and around 70 DWRs.
- Underutilized data from ground stations: Although state governments and private companies manage over 20,000 ground stations, much of this data is inaccessible to the India Meteorological Department (IMD) due to issues with data-sharing and reliability.
- Difficulty in predicting small-scale events: Large systems like monsoons or cyclones are easier to forecast, but sudden, localized events like cloudbursts remain challenging due to their erratic and dynamic nature.
- Increasing climate volatility leads to frequent and rapid system changes, complicating predictions even further.
Key initiatives taken to facilitate hyperlocal weather forecasting
|
Conclusion
India needs a comprehensive approach to enhance hyperlocal weather forecasting. This includes upgrading to advanced models, expanding the monitoring network, fostering data-sharing, and developing robust real-time data systems. By addressing these areas, India can improve accuracy, especially for localized events, and better prepare for extreme weather.